In this modern era, people perceive financial algorithms as witchcraft because they don’t understand the first thing about it. Even the frontline lenders’ staff do not know how algorithms decide fundability™ or funding approvals. However, it’s been proven over and over again that technological advances are not necessarily evil. In this episode, Merrill Chandler talks about the things we need to understand about algorithms and what they are designed for. He also tackles Goldman Sachs’ alleged gender bias in Apple credit card limits.
—
Watch the episode here
Listen to the podcast here
Merrill’s Rant: A Modern Day Witch Hunt
This whole Apple credit card, Goldman Sachs, the whole idea of algorithms being designed with gender bias, even with socioeconomic bias, that’s a burr under my saddle. We’re going to talk about this and we’re going to challenge everything. If you haven’t read my previous blogs, you’ve got to know I am not an apologist for FICO. I’m not an apologist for lenders. I’m not an apologist for borrowers. I want to suss out the truth and find out what’s going on. Usually, the truth is in the middle ground. The first thing that I want to share is that the vast majority of what’s going on looks like a freaking witch hunt.
Are Financial Algorithms Withcraft?
People act like financial algorithms are witchcraft. It goes back to that ugly part of the human lizard brain that says, “If I don’t understand it, it’s evil.” It’s the thing we’ve proven over the last 1,000 years. We’ve proven over and over again that technological advances are not necessarily evil. If we don’t understand it, it doesn’t make it evil. In the Spanish Inquisition, they didn’t understand the whole idea of ecstatic spiritual experiences. “We have to kill them all because what we don’t understand is evil.” I call it crap, this whole thing. It’s like finding the algorithms among the vast majority. Even the frontline lenders staff does not know how algorithms decide fundability™ or funding approvals. It’s like if a medieval serf found a flashlight and was like, “Look at this thing. I pushed the button and the light comes on.” They didn’t understand it. That person would probably have been burned at the stake because it’s not intelligible within the current paradigm of understanding and that makes it evil.
Follow The Money
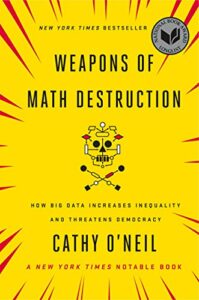
Weapons of Math Destruction: How Big Data Increases Inequality and Threatens Democracy
I’m going to take both sides of this argument, but let’s walk through this. First, follow the money. That is the number one thing in everything we do, especially in the financial world. Follow the money and you’ll find the motivations and opportunities. I‘ll ask the perennial question that I ask every bootcamp, in my book 100 times and on all of my shows, “When do lenders make money?” They make money when they lend. That’s why we call them lenders. If they make money when they lend, what does it serve? A smoke-filled room of white men who are all smoking cigars with their brandy snifters like every cabal movie on the planet ascribes to the privileged white middle or 1%. If that’s true, what do they win by not lending to women, to minorities or to whomever? They don’t win anything.
They make money when they lend. We’ve got to follow the money. Let’s take a look at this. I did a bunch of research, read several articles, and I have one Q and A. The Twitter thread was done by David Hanson. He is a well-known software engineer. He posts regularly on his Twitter account. All of a sudden, the author of this interview spoke to Cathy O’Neil. She’s a mathematician and an author of a book. I love the name of this book. It’s called Weapons of Math Destruction: How Big Data Increases Inequality and Threatens Democracy. I don’t necessarily agree with the premise that the title of her book conveys. What I do want to do is ask in this show the same question she was asked by the writer of this article.
In this article, she calls it straight up. At first glance, it seems like a pretty convincing case of algorithm fueled gender discrimination. She says in the first sentence, “We don’t have enough information to know what is going on here. The truth is, people have all sorts of data that we don’t even know about in our profiles. If they’re not accurate, they’re available to corporations like Apple.” We’ve been talking about this for generations. Many people reacting to this stuff are not Bootcamp grads. They haven’t read my book. They don’t know that there is a huge automated underwriting universe that pulls out data from numerous databases, grades, all of that data and gives us a funding decision or not.
What Algorithms Are Designed For
Here’s the fascinating thing. She’s right on. We don’t have enough information about this, but one of the things we need to understand is that the algorithms are designed to do two things. One, it’s designed to protect the lender money by giving that money to someone they trust will treat it well. They will keep their agreements and pay it back. The number one goal of all automated underwriting systems is how do we use the data, use the statistics of success and failure and find out what is the demographic? What is the person by person? It doesn’t matter if you’re part of a group or not, your data is measured. Once that data is measured, you’re given a lending decision approved at the requested amount, approved at a lower amount or denied.
Let’s go back to what was said that David Hanson was given twenty times higher of a limit than his wife. We don’t know from any of their data when they filed and if they filed jointly or did they file separately. Is it a joint credit card? If it’s a joint credit card, it would have been one limit that they both share. We can presume that they filed separate applications. What we also don’t know is that Jamie, the wife says, “I’ve had credit in the US far longer than David. I’ve never had a single late payment. I do not have any debts. David and I share all financial accounts and my very good credit score is higher than David’s.” Do you see any questions to ask Jamie and any person who’s automatically going to gender bias here? You guys are all funding hackers. You guys are all insiders. Do you see any problems with her quote? She’s got a better average than David, but we don’t know what she means by credit. Is it secured credit? Is the mortgage in her name? How long has she had credit? Is it unsecured credit cards? It’s like lending. The lenders are not looking at your car loan and your mortgage history when they’re evaluating how you treat unsecured credit cards. We know this. She says, “I’ve had it far longer than David.” What does she have? Did she have a mortgage back in the day? She had student loans for twenty years. Those are relevant to today’s approvals.
Women are the single largest demographic who are engaging in credit #GetFundable Click To TweetThe next thing she said is, “I’ve never had a single late payment.” Remember how I complain when I go out in the world and everybody is like, “Why can’t I get any funding? I’ve never been late.” You guys know this, what is my automatic response to anybody who says, “Why can’t I get funding? I’ve never been late.” What about the other 39 metrics that FICO measures? Paying on time keeps you in the game. Being late benches your ass. She’s saying that she’s relying on late payment as the only metric of why her husband got twenty times more. The next one she says is, “I do not have any debts.” What is the most recent history? Is he running traffic on his credit, but she doesn’t run any traffic on her accounts? How many opened accounts are there? She went for a co-branded Goldman Sachs, a tier two institution. It’s co-branded, so it’s 80%. With Apple, what does her husband have in his revolving accounts portfolio? What are the limits that his husband has? If she has equivalent limits, then that’s one of the things that we would look at. She says, “I don’t have any debt.” If she doesn’t have a mortgage, doesn’t have an auto loan, doesn’t have regular ongoing traffic into this experience, what is she going to do?
Having no debt, remember FICO and lender software are like, “What have you done for me lately?” Finally, there’s, “David and I share all financial.” Checking accounts, that’s a marginal contribution to the FICO score. “My very good credit score is higher than David’s,” but the score is not what is accounting here. It’s all of the other metrics. We’ve got to figure out the same with Apple Cofounder Steve Wozniak, who got it ten times higher than his wife, but there are so many other metrics that need to be measured to see if this is gender-specific, if it’s gender-biased. I have this metaphor and the metaphor is a little nonsensical, but that’s where I want to go with it. Let me use this metaphor. With as much logic, someone can say, “Why is the sky not green? It is color bias to blue. I want to know why the sky is not green. There are many other colors that it could be, but why is it blue? There is bias in the universe for creating this result called a blue sky.” For those of us who are aware of those things, light filtering through the ozone, light filtering through the stratosphere, and all of the physics, all of the chemistry of light going through our atmosphere creates a blue sky. Someone could easily say, “This is crap. The sky, the universe is biased to blue skies.” That argument is nonsensical, but it’s based on the same principles that the algorithm is gender-biased because all of the math is based on what protects lender money.
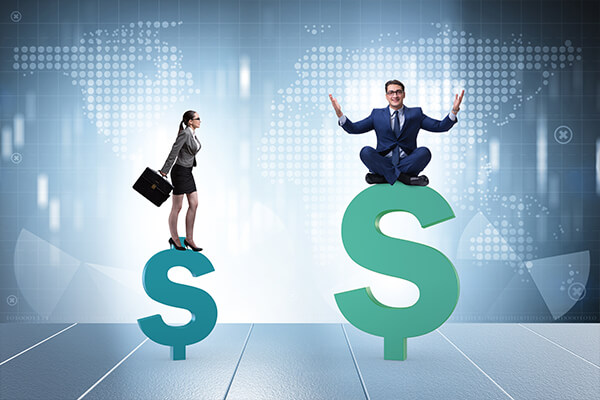
Financial Algorithms: Women are making the financial decisions to a significant degree. Lenders would be foolish to bias against the people with the greatest successful demographic.
The Largest Successful Demographic
Just so you know, the word is out. in 2017, 65% of all mortgages were done by women. Up to 80% of all financial debt engagement are women. These are the bank rate and comptroller of the currency statistics. Up to 80%, depending on what you’re defining as revolving installment, of all credit engagement is done by women. They lead households or in charge as part of separating financial duties between husband and wife. We need to ask harder questions. I’m an Apple-phile. I’m a FICO-phile. I’ve got as many complaints against lenders as the next guy. In fact, I have something here that I want to tell you. It’s from FICO World. We’re going to be doing deeper dives into FICO World. American Banker did a survey for FICO asking all executives, and the number of them was huge. There were several hundred detailed responses from senior experts.
One of the things that they did ask, which was the perfect time for this supposed controversy is they asked that in simulating and validating strategies, many companies make important strategic decisions without first simulating them to understand possible outcomes and unintended consequences. The takeaway is that fintechs believe that they were extremely confident about doing tests to see what the outcomes of these modeling situations in this automated underwriting software would be. Traditional banks were at 5%. They were extremely confident in their ability to simulate alternative outcomes so that they could see what the results would be. Goldman Sachs is moving into personal and business lending, but it would be classified as a fintech. I don’t know what their answers were, but if they fall into that 20%, they’re only 20% confident of testing their software to see what the outcomes would be. I’m not saying testing the software is going to show a gender bias. Lenders want to lend. If somewhere between 65% and 80% of women are making the financial engagements, they’re the ones applying for credit, why would lenders want to discriminate against women?
They’re the single largest demographic who are engaging credit. Algorithms are witchcraft. This is one of my Merrillisms. Later on, I’m producing a book of all of my Merrillisms that does a deep dive into every one of these. One of the things that I learned a long time ago is that we are not critical enough. We are critical to engage in argument, to support our comfort zone and our beliefs about something. If somebody walks around filtering their lives for gender bias or socioeconomic bias, they’re going to find socioeconomic bias everywhere they go. I’m going to stand by this. I acknowledge that there is a socioeconomic bias. I acknowledged that there is gender bias, but do you know where that bias comes from? It’s meeting one-on-one with bankers in the branches trying to engage credit.
The socioeconomic bias and the racial bias is in the humans being involved, not the math #GetFundable Click To TweetHumans are biased. The math is not. I will eat my words. You will see me right here in a week, a month, a year, whatever it is, where somebody comes up and they prove that there was a gender bias in this software. I will apologize profusely and I will say, “That one, I got wrong.” Pay attention to my argument. Are we critical enough? The gender bias is in the human engagement of these loans. The socioeconomic bias, the racial bias is in the humans being involved, not the math. The math is designed to make profitable decisions with everybody regardless of race, creed, color, gender or sexual preference. It’s designed to make money. Depending on the metric you’re measuring, 65% to 80% of financial credit engagements are done by women. They are not going to push that demographic away by biasing against them. That’s insanity.
Is the answer done? We don’t know. There have been great responses out there that without giving up the IP, you can do testing. In the upcoming episodes, we’re going to be talking about a number of subjects that we got from FICO in this FICO world that show brand new scoring models. I’m telling you, these scoring models are designed to protect lender income or lender resources. It’s also designed to move all of the decisions away from biased human beings. One of them is called the FSSI or the Financial Score Stress Indicator. It’s designed to protect lenders.
When I say protect lender money, you need to hear it the exact same time, “Give me an opportunity to get funding because I know the rules of how to get a yes.” That’s what these algorithms mean. Please forgive me if you’re incensed by this. You don’t even have to agree. It’s not witchcraft. It’s beyond the comprehension of most of us, including me. I don’t know how to read an algorithm, trail logic, data lakes and decision trees that lead to decision tree forests. I barely comprehend some of this stuff. What I do comprehend through and through is lender profit motive. That I got dialed. Don’t get lost in the BS that’s going on out there trying to create and make a pariah of lending decisions when someone who they themselves say, “I don’t have any debts.”
You’re going to get a test limit. If you don’t have any debts, you’ve never made a late payment and you share financial accounts, she does not know what makes her fundable. Until I get his and her MyFICO.com/creditprofile and put them together, then I’m not going to get my undies in a bungee because it’s biased towards profitability. Women are making the financial decisions to a significant degree and the lenders would be foolish to bias against the people with the greatest successful demographic. Most of the stuff is we don’t have enough information. There are two types of evidence that we would want, like some statistical evidence about fairness. They talk about fairness. Fairness is not giving everybody money equally, but that’s what it says. They would have to define what it means to be fair with respect to gender, fair with respect to race, or fair with respect to veterans’ status, or whatever the protected class is. That very thing is that algorithm is designed to not play that game.
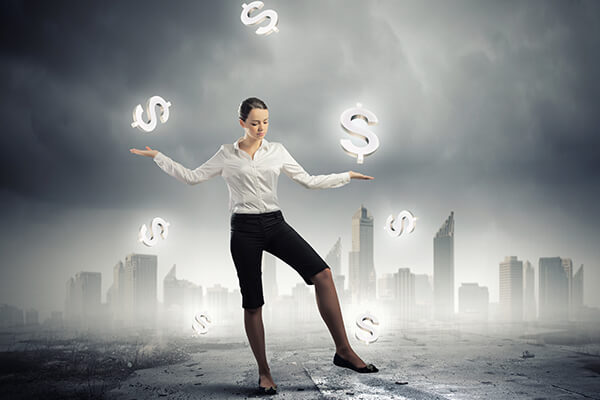
Financial Algorithms: Income and the ability to pay drive the lower limit; it’s math.
Zip Codes And Revenue Scores
Some people can argue, “Merrill, you tell us in the Bootcamp that there is a revenue score.” The revenue score is designed to create a score that tells a lender how much money they can anticipate from a particular score and a particular zip code. The zip code is designed. There are zip codes where there are only African-Americans. There are Latin zip codes. There are Asian zip codes. There are Caucasian zip codes, and there are WASP zip codes, White Anglo-Saxon Protestant older male, one-percenters. Even the revenue score, what their score is and how much will they make depends on their income. They’re in the zip code. Generally speaking, most people reside in a zip code because that is the socio-economic location of their wages, income and compensation. If they have a good score, they very well may be trustworthy.
They’re firing on all of the qualification metrics. They’re going to make money, but they may not get as much of a limit because there’s only so much money that is disposable income. The income drives the lower limit. The ability to pay drives the lower limit. It’s math. Your race doesn’t drive your lower limit or higher limit. It’s your income and it is covered by Falcon and all the application fraud management mechanisms that FICO and the lenders use. We’re going to have another episode on that. It’s not where you live. It is that where you live, not always, I’m only speaking in general, reasonably is an indicator of your ability or your revenue. You live in that zip code because that’s the type of home, apartment, housing that you can afford. That’s based on revenues. Is there gender bias on salaries? All of the studies are in that there is a significant bias, but that bias is not driven by hiring algorithms. That bias is men in power, women in power are looking at women. I heard both sides of this argument. Men discriminate against women in the salary process.
The math is designed to make profitable decisions with everybody regardless of race, creed, color, gender or sexual preference #GetFundable Click To Tweet
Other women discriminate against women because they said, “You’ve got to earn your chops as I did.” Whatever the reason, it’s human bias, not the algorithm. If we did hire through algorithms, we’d probably have way less, if not, completely remove the wage bias. Let’s say, hiring metrics had 40 calculations like FICO. I bet you there would be no bias in hiring anymore. I’m willing to be wrong, but when you follow the math and you follow human nature for the last zillion years that what you don’t understand is evil, then the algorithm, the automated underwriting systems are just a flashlight in medieval Europe. We will all come to understand this over time. There will be more modeling where it will become more consumer-friendly and borrower-friendly. This is a great forum. Thanks for reading this. I hope you made it to the end because I’m biased against bias. It’s not the math that’s biased. It’s us humans. Have a spectacular rest of your day. The most powerful way to become fundable is to optimize your borrower behaviors in alignment with these very algorithms and models. We’ll continue to be successful beyond every person who doesn’t.
Important Links:
- Weapons of Math Destruction: How Big Data Increases Inequality and Threatens Democracy
- FICO World
- MyFICO.com/creditprofile